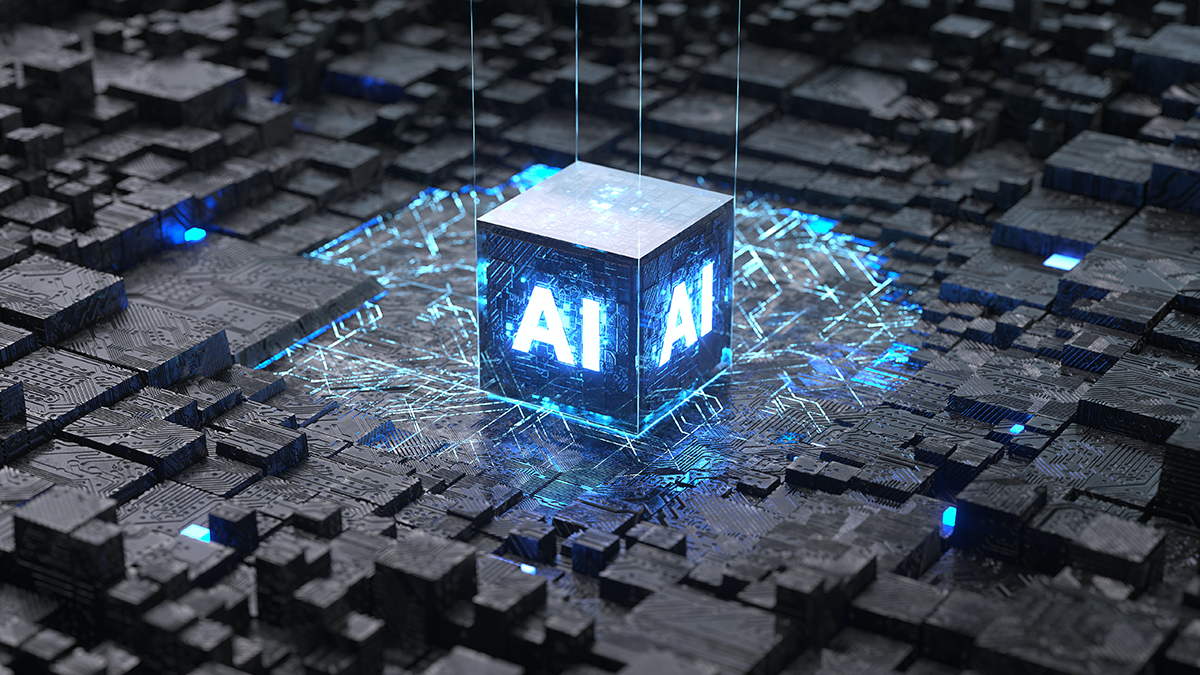
11 Aug AI’s potential to improve clinical operations, trial matching
By Tyler Menichiello, Clinical Leader
These days, it seems like nothing is as widely discussed (positively or negatively) as AI. Good or bad, the ball will continue rolling, so it’s important to dive head-first into the conversation. With the rise of generative AI models like ChatGPT, there come questions of how this technology can be used in industry, especially in healthcare. At this year’s DIA conference, Dr. Hoifung Poon, general manager of Microsoft Health Futures, spoke about AI’s potential to improve efficiency in healthcare and the life sciences industry. Though I didn’t attend DIA, I was lucky enough to speak to Dr. Poon about his work developing large-language models (LLM) for use in biomedical research. He says AI can be used in clinical research to improve patient care by improving clinical workflows and making it easier to match patients to trials.
Superhuman Curators
Simply put, LLMs are AI models capable of understanding language and generating text. The best way to think about it is like a predictive text software on steroids or a sophisticated form of autocomplete. The “large” in the name refers to both the size of these systems’ complicated networks, as well as the huge datasets that these models are trained on to understand human language. “The way you actually train these models is essentially by playing hide and seek with words,” Poon explains. “Then, you ask the model to predict them using context.” By training these neural networks on tons of medical text (available on the public web), LLMs are becoming increasingly more capable of understanding and structuring textual data. Poon’s team is working to harness these emergent capabilities for “structuring medical data at scale.”
“What we really want is a data-driven, continuously learning health system where any new piece of information about a patient, trial, or drug can instantly feed back into the process.” The main challenge in achieving this is that medical data is largely unstructured. Unstructured data essentially refers to anything written or described in natural language, such as the information contained in a doctor’s note. “The reason they’re unstructured is because there’s a lot of variation,” Poon explains. For example, take type 2 diabetes, which can be referred to as type 2 diabetes mellitus or adult-onset diabetes. There’s also a lot of variation and ambiguity among medical acronyms, which can often have multiple meanings (e.g., ER and PDF). Structured data, on the other hand, is like the information in a database or numbers in a spreadsheet — it’s organized and neatly gathered in one place. Read more …